Data Observability
In the era of big data and advanced analytics, organizations are increasingly relying on data to drive decision-making processes. However, the complexity and scale of data ecosystems have made it challenging to ensure data reliability, accuracy, and performance. This is where data observability comes into play. Data observability enables organizations to gain deeper insights into their data pipelines, ensuring data quality, integrity, and observability across the entire data lifecycle.
What is Data Observability?
Data observability refers to the practice of monitoring, understanding, and improving the reliability, quality, and performance of data pipelines. It involves the collection, analysis, and visualization of data-related metrics, logs, and events to ensure the availability, correctness, and transparency of data.
The Five Pillars of Data Observability
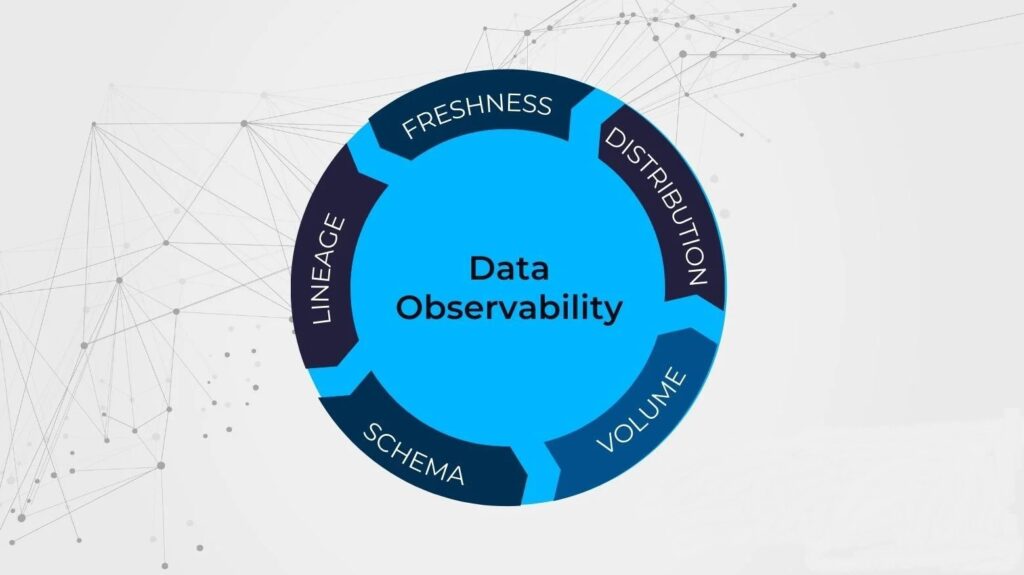
1.Freshness: Freshness refers to the timeliness and currency of data. It focuses on ensuring that data is up-to-date and reflects the most recent information available. Monitoring data freshness involves tracking data arrival times, latency, and delays in data processing to identify any potential issues that could impact real-time decision-making or analytics.
2.Quality : Data quality is a critical pillar of data observability. It emphasizes the accuracy, completeness, consistency, and reliability of data. Data quality monitoring involves assessing and validating data against predefined standards, business rules, or quality metrics. By continuously monitoring data quality, organizations can identify and rectify data issues or anomalies, ensuring trustworthy and reliable data.
3.Volume: Volume refers to the scale and magnitude of data. Data observability considers monitoring data volume as a crucial pillar to ensure that the infrastructure and systems can handle the expected volume of data. Monitoring data volume involves tracking data ingestion rates, storage capacity, and resource utilization to ensure the smooth functioning of data pipelines and prevent performance bottlenecks.
4.Schema : Schema refers to the structure, format, and organization of data. It focuses on ensuring that data adheres to predefined schemas or data models. Monitoring data schema involves validating data against schema definitions, detecting schema changes or inconsistencies, and ensuring data compatibility across different systems or applications.
5.Lineage : Lineage pertains to understanding the origin, transformations, and flow of data throughout the data pipeline. It involves tracking and documenting the journey of data from its source to its destination, including any transformations or modifications it undergoes. Monitoring data lineage enables organizations to trace data dependencies, track data changes, and ensure data accuracy, compliance, and audibility.
Why is Data Observability Important?
Early Issue Detection: Data observability enables organizations to identify and address data issues proactively. By continuously monitoring data pipelines, potential problems can be detected before they impact critical processes or decision-making.
Enhanced Data Reliability: With data observability, organizations can have confidence in the accuracy and quality of their data. It helps in reducing data-related errors and ensures data consistency, which leads to better decision-making and improved operational efficiency.
Improved Data Pipeline Performance: By monitoring data pipeline performance, organizations can identify bottlenecks, optimize resource utilization, and improve overall data processing efficiency. This results in faster insights and improved productivity.
Compliance and Risk Management: Data observability helps organizations meet regulatory requirements and manage data-related risks effectively. It ensures adherence to data privacy, security, and governance standards, thereby protecting sensitive information and mitigating compliance risks.
Benefits of Data Observability
Reliable Decision Making: Data observability provides organizations with reliable and trustworthy data, enabling data-driven decision making with greater confidence and accuracy.
Increased Operational Efficiency: By identifying and addressing data issues promptly, data observability helps streamline operations, reduce downtime, and minimize the impact of data-related disruptions.
Enhanced Customer Experience: Improved data quality and performance lead to better customer experiences, as organizations can deliver personalized and relevant services based on reliable data insights.
Cost Optimization: Data observability helps identify inefficiencies in data pipelines, allowing organizations to optimize resource allocation, minimize unnecessary costs, and improve return on investment.
Data Observability vs. Data Monitoring
While data monitoring focuses on tracking the status and availability of data systems and processes, data observability goes beyond simple monitoring. Data observability provides deeper visibility into data pipelines by capturing and analyzing relevant metrics, logs, and events. It offers insights into the quality, integrity, performance, dependencies, and compliance of data, enabling proactive issue detection and comprehensive data management.
Key Features of Data Observability Tools
Metrics and Monitoring: Data observability tools provide real-time visibility into data pipelines by collecting and analyzing metrics such as data latency, error rates, and throughput. They enable proactive monitoring and alerting of potential issues.
Data Validation and Auditing: These tools offer capabilities to validate data integrity, accuracy, and consistency throughout the data lifecycle. They help identify and rectify anomalies or discrepancies in data.
Visualization and Dashboards: Data observability tools provide intuitive visualizations and customizable dashboards to display key metrics and insights. They enable users to monitor data health, identify trends, and investigate issues effectively.
Anomaly Detection: These tools employ machine learning techniques to detect abnormal patterns or deviations in data pipelines. They can automatically identify outliers, data drift, or unexpected behavior and trigger alerts for further investigation.
Data Tracing and Dependency Mapping: Data observability tools enable organizations to understand data lineage, track data dependencies, and visualize data flows across different systems and processes. This helps in identifying bottlenecks, optimizing workflows, and ensuring data consistency.
Conclusion
In the data-driven world, data observability has emerged as a crucial practice to ensure the reliability, quality, and performance of data pipelines. By focusing on data quality, integrity, performance, dependencies, and compliance, organizations can unlock the true potential of their data, make informed decisions, and drive business success with confidence. Adopting data observability tools and practices equips organizations with the necessary visibility and control over their data ecosystems, empowering them to thrive in a data-centric future.