Introduction to Data Lakehouse
A data platform known as a “data lakehouse” combines the finest features of data lakes and warehouses into a single data management solution. Although data warehouses can be more expensive and have fewer scalability options than data lakes, they are typically more performant than the latter. By using cloud object storage to store a wider variety of data kinds, including structured, unstructured, and semi-structured data, a data lakehouse aims to address this problem. Data teams can process data more quickly by consolidating these advantages under a single data architecture because they are no longer need to work across two dissimilar data architectures.
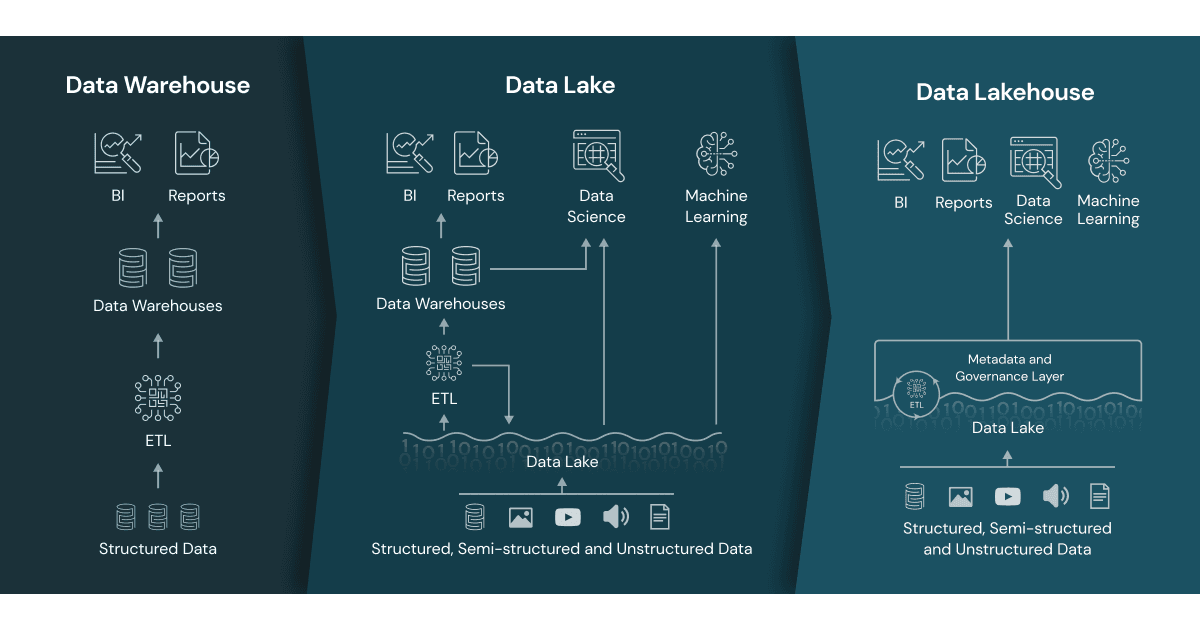
Benefits of a data lakehouse:
Organisations can create a single data platform by creating a data lakehouse, which will streamline their overall data management process. By dismantling the barriers between various sources, a data lakehouse can replace the need for distinct solutions. Compared to curated data sources, this integration produces a significantly more effective end-to-end procedure.
Reduced data redundancy: A simplified platform to handle all company data demands is made possible by the single data storage system. By minimising the amount of data flowing via the data pipelines into various systems, data lakehouses also make data observability simpler.
Cost-effective: The operational costs of a data lakehouse are significantly lower than those of a data warehouse because it takes use of the cheaper cloud object storage. A data lakehouse’s hybrid architecture also reduces operating costs by doing away with the need to maintain several data storage systems.
Supports wide variety of workloads: Different use cases can be addressed by data lakehouses throughout the data management lifecycle. Additionally, it may support more sophisticated data science workstreams as well as business intelligence and data visualisation ones.
Better governance: The typical governance problems associated with data lakes are reduced by the data lakehouse architecture. For instance, it can make sure that the data fits the established schema criteria as it is ingested and uploaded, preventing difficulties with downstream data quality.
More scale: Coupled computing and storage in classic data warehouses increased operational costs. The ability for data teams to use the same data storage while using different computing nodes for various applications is made possible by data lakehouses, which segregate storage and computation. More scalability and flexibility emerge from this.
Streaming support: Many data sources use real-time streaming directly from devices since the data lakehouse is designed for today’s business and technology. This real-time ingestion is supported by the lakehouse system and is expected to grow in popularity over time.
Features of a Data Lakehouse:
Data Management: Data management capabilities like data cleansing, ETL, and schema enforcement are frequently provided by a data warehouse. These are added to a data lakehouse in order to prepare data quickly, allowing data from selected sources to naturally interact with one another and be ready for additional analytics and business intelligence (BI) tools.
Open Storage Formats: Using open and standardized storage formats means that data from curated data sources have a significant head start in being able to work together and be ready for analytics or reporting.
Flexible Storage: The ability to separate compute from storage resources makes it easy to scale storage as necessary.
Support for Streaming: Real-time streaming directly from devices is used by several data sources. In comparison to a typical data warehouse, a data lakehouse is designed to facilitate this kind of real-time intake better. Real-time support is vital since the Internet of Things is integrating the globe more and more.
Diverse Workloads: A data lakehouse is the perfect answer for a variety of workloads since it combines the advantages of a data warehouse and a data lake. The intrinsic properties of a data lakehouse can support a variety of workloads within an organisation, including business reporting, data science teams, and analytics tools.
Conclusion:
Data lakehouses have become an effective and adaptable way to combine data lakes and data warehouses. Organisations may create a unified, scalable, and affordable data platform that enables real-time analytics, streamlines data management, and improves data governance by leveraging the advantages of both designs. In today’s data-centric environment, adopting the data lakehouse approach can enable firms to maximise the value of their data and obtain a competitive advantage.
A data lakehouse implementation involves thorough design, taking into account the unique business needs and technical ecosystem. However, the advantages it provides make it an appealing option for businesses looking to maximise the value of their data assets.